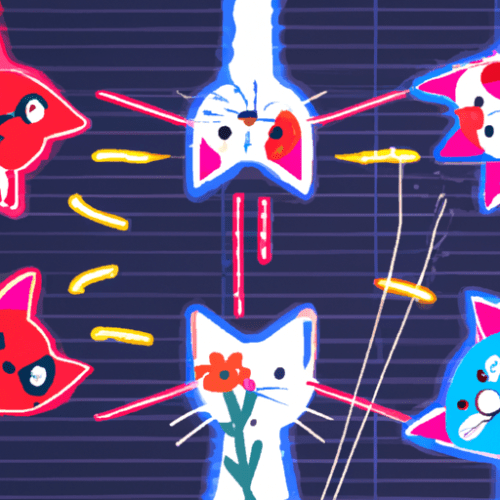
In the rapidly transforming landscape of localization and global communication, the translation memory (TM) has long stood as one of the cornerstones of efficiency and quality. Since its inception, translation memory technology has evolved from rudimentary, static databases into sophisticated, adaptive systems capable of predictive, context-sensitive assistance. This article offers a detailed perspective on the evolutionary path of translation memory, exploring its origins, transformations, and its emerging role as an intelligent, integral component in the broader ecosystem of translation technology. Experienced translation and localization professionals will gain a deeper understanding of TM’s trajectory and its promise for the future.
Introduction to Translation Memory: Foundational Concepts
A translation memory is, at its core, a linguistic database that stores segments of translated text—usually sentences or sentence-like units—which can later be reused to improve translation speed, consistency, and cost-effectiveness. While Computer-Assisted Translation (CAT) tools often incorporate many sub-technologies, TM stands out for its direct impact on the translator’s productivity and the client’s savings, especially in projects with substantial repetitions or updates. Historically, TM was designed as a “match and retrieve” tool, retrieving stored translations based on fuzzy or exact matches with new source content.
Over the past 30 years, the technological and conceptual underpinnings of TM have matured at an unprecedented pace. The contemporary market is witnessing an inflection point: TM systems are moving beyond static retrieval to embrace dynamic, AI-driven approaches that promise not just to remember, but to learn and predict.
The Static TM Era: Advantages and Limitations
The initial implementations of translation memory focused on the static storage of segment pairs (source and target), typically relying on string-based matching algorithms. These early systems, prominent in the 1990s and early 2000s with providers such as Trados and SDLX, delivered major advancements in productivity for sectors dealing with highly repetitive content, such as software documentation, legal contracts, and technical manuals.
Key benefits of static TM systems:
- Consistency: Reuse of previous translations enforced terminological and stylistic uniformity.
- Productivity: Translators no longer needed to retranslate identical or similar content, saving substantial time.
- Cost efficiency: Clients benefited from reduced translation costs thanks to discounts on repeated segments.
Despite these advantages, static TM’s approach soon revealed significant limitations:
- Context blindness: Early TM systems operated almost exclusively at the segment level, disregarding broader textual context and leading to inappropriate or awkward translations in ambiguous segments.
- Lack of adaptability: Static TMs could not dynamically adjust to changing linguistic trends, domain shifts, or personalized preferences. All translation matches were “as stored”; mistakes were perpetuated unless manually corrected.
- Maintenance difficulties: Over time, large TMs became unwieldy, with issues of duplication, outdated translations, and a need for frequent, labor-intensive cleanup.
The Rise of Dynamic and Contextual Translation Memory
The limitations of static systems prompted both technology vendors and translation professionals to demand more powerful, flexible TM solutions. The last decade has thus seen the emergence of dynamic TM systems. These leverage advances in computational linguistics, database architecture, and, most importantly, artificial intelligence.
Key features of new-generation dynamic TMs include:
- Context-aware retrieval: Modern TM systems utilize linguistic/structural cues—such as document structure, previous/following segments, and metadata—to offer contextually appropriate matches. This reduces the risk of misleading repetitions.
- Automated curation: AI-driven tools can now flag, de-duplicate, or suggest corrections to stored segments, greatly reducing manual maintenance.
- Collaborative and cloud-based infrastructures: Multi-user, real-time TMs hosted in the cloud enable organizations to harness global translation resources, even integrating contributions from crowdsourcing or machine-generated post-editing.
- Fine-grained control and segmentation: The segment is no longer static; advanced segmentation respects linguistic boundaries (such as clauses and sub-sentences) for more granular memory building.
Some platforms have even begun to offer adaptive translation memories: systems that learn from the translator’s real-time choices and provide suggestions accordingly. Examples include SDL’s AdaptiveMT functionality and MemoQ’s LiveDocs, which integrate multiple sources—including machine translation, legacy TMs, and human feedback—into a seamless, constantly learning environment.
The Predictive, AI-Driven Paradigm in Translation Memory
The most transformative development in translation memory lies in its fusion with predictive, neural-network-based AI. While TM was previously limited to segment retrieval, today’s systems are beginning to exploit vast translation datasets and sophisticated model architectures to predict the best possible translation—or even suggest entire segments that never existed in the database.
Machine Translation Post-Editing (MTPE) workflows, which were traditionally considered distinct from TM-based processes, are becoming ever-more entwined. Predictive TM systems, now powered by AI, blend the strengths of both: leveraging both human-vetted memories and live machine hypotheses to ensure maximum quality and efficiency.
Furthermore, leading-edge TM integrations now offer:
- Predictive typing and autocompletion: Similar to email autocomplete, translators receive segment suggestions as they type, drawn from both TM and MT sources, but tuned to the current project’s lexicon and style.
- Domain adaptation: Intelligent systems automatically tailor suggestions based on content type—be it legal, medical, or marketing—by identifying style, register, and terminology from past translations and external corpora.
- Quality scoring and feedback loops: The system scores suggested translations according to likely accuracy, consistency, and fluency, allowing for immediate user review and incremental improvement of memory stores.
- Integration with translation analytics: Real-time dashboards enable project managers to assess TM utilization rates, identify bottlenecks, and optimize linguistic resource allocation.
With advances in Deep Learning, translation memory is poised to become even smarter. Predictive TM systems can learn the stylistic and terminological preferences of individual clients or industries, adapting on the fly and offering a blend of automation and human touch that neither TM nor MT alone could ever achieve.
Challenges and Considerations
Despite these impressive advances, the new era of TM presents its own challenges:
- Data privacy: Cloud-based, collaborative memories require rigorous security to protect intellectual property and client confidentiality.
- Transparency and explainability: As predictive TM systems become more “black box,” translators and clients must remain vigilant against errors and ensure that AI-driven suggestions meet required quality standards.
- Alignment and trust: The increasing sophistication of memory systems necessitates retraining and buy-in from linguists, project managers, and technologists alike.
Nonetheless, the trajectory is clear: only those organizations who embrace and skillfully leverage these new TM paradigms will remain competitive in the accelerating global content economy.
Conclusion: The Future of Translation Memory
The evolution of translation memory from simple, static repositories to dynamic, intelligent systems marks a paradigm shift in the linguistics industry. What began as a tool for basic reuse and consistency is emerging as an ecosystem that combines human expertise, scalable automation, and intelligent prediction. As predictive, context-sensitive TMs proliferate, the expectations for translation quality, speed, and customization will continue to rise.
For translation and localization professionals, embracing these new capabilities is not just a matter of productivity—it is vital for ensuring relevance and excellence in a digital world that demands instant, accurate, and culturally attuned communication. As TM merges seamlessly with the broader constellation of AI-driven tools, its evolution is set to continue: increasingly adaptive, predictive, and indispensable to organizations seeking to make their voices heard across every language and market.
The collaborative dance between human translators and intelligent systems will define the next decade of global communication. Those who master the orchestration of static knowledge and dynamic prediction will set new benchmarks for what is possible in translation—and in connection.
#translation memory #evolution of translation memory #translation technology #dynamic translation memory #predictive translation memory #AI translation memory #translation memory systems #CAT tools #computer-assisted translation #localization #translation memory database #translation memory benefits #adaptive translation memory #context-aware translation #translation productivity #TM systems #static translation memory #neural machine translation #machine translation post-editing #memoQ #Trados #SDLX #transla