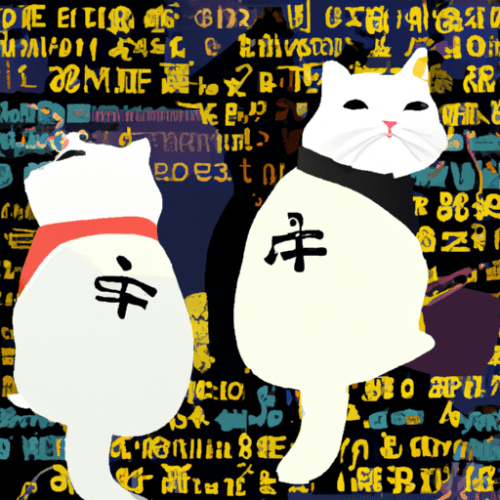
The translation and localization industry has long been characterized by the dynamic interplay between human expertise and evolving technological advancements. In the past decade, Machine Translation Post-Editing (MTPE) established itself as a mainstream workflow for accelerating translation output and reducing costs. However, with the emergence of Large Language Models (LLMs) such as OpenAI’s GPT-4 and Google’s PaLM, a new paradigm is quickly gaining traction: Prompt-Editing. This article delivers a high-level analysis of these two approaches — Post-Editing with legacy MT systems and Prompt-Editing with LLM-powered solutions — outlining their respective strengths, challenges, and the profound impact LLMs are having on the linguist’s workflow and the wider localization landscape.
Introduction: Evolving Approaches to Translation Quality
For decades, the concept of post-editing has been fundamental to the integration of machine translation into production workflows. In this model, a neural machine translation (NMT) system delivers a first draft that is then meticulously refined by human linguists. While this process has undoubtedly improved efficiency, it has also revealed persistent friction points, such as repetitive errors, productivity plateaus, and the challenge of maintaining stylistic and terminological consistency across large-scale projects.
Enter Prompt-Editing: the practice of iteratively refining LLM output by optimizing the prompts fed to these advanced models, either preemptively (prior to content generation) or reactively (after reviewing initial output). This technique leverages the contextual and adaptable nature of LLMs, transforming them from static translation engines into dynamic assistants capable of nuanced, context-aware translation and localization.
The Traditional Model: Post-Editing Machine Translation
Post-editing has played a pivotal role in enabling the mainstream acceptance of machine translation. The typical workflow comprises:
- Running source content through an NMT system (e.g., Google Translate, DeepL).
- Assigning linguists or editors to review and correct output, ensuring accuracy, fluency, and adherence to client guidelines.
- Delivering edited translations for publication or further localization.
Advantages: This approach delivers clear productivity benefits, lowers costs, and enables the rapid translation of large volumes of content while keeping human expertise in the quality assurance loop.
However, post-editing is not without drawbacks:
- Repetitive Corrections: Systematic errors (e.g., terminology mismatches, grammatical inconsistencies) require manual correction with every iteration if not captured in post-editing guidelines or glossaries.
- Cognitive Load: The task resembles “polishing” text rather than creating new content, which can undermine engagement and job satisfaction for linguists.
- Customization Limitations: Adjusting the translation style or tone often requires manual intervention or complex configuration of engine glossaries or custom models — a process that is both time-consuming and technically challenging.
The New Paradigm: Prompt-Editing with LLMs
LLMs have revolutionized text generation and translation by understanding and generating human-like text based on sophisticated prompts. With this advance comes Prompt-Editing, a fundamentally different approach:
- Initial Prompt Design: Crafting detailed prompts that encapsulate not only the translation request but also contextual information, preferred terminology, tone of voice, and client-specific requirements.
- Iterative Prompt Refinement: Upon reviewing LLM output, linguists modify prompts to address any detected issues — be it style, register, accuracy, or context misinterpretation — and quickly regenerate improved translations.
- Extensive Customization: As LLMs are highly context-sensitive, even minor prompt adjustments can yield significant shifts in output quality and alignment with project goals.
Advantages of Prompt-Editing:
- Fewer Manual Corrections: Systematic translation patterns can be “trained” on the fly by adjusting prompts, reducing repetitive error correction over time.
- Greater Flexibility: Linguists can specify requirements (e.g., maintain brand voice, use region-specific language, avoid sensitive terms) that are executed immediately, often without in-depth technical knowledge or system reconfiguration.
- Higher Linguistic Quality Potential: LLMs excel at preserving nuance, syntactic structures, and stylistic elements when properly guided.
- Time Savings: Iterative prompt refinement frequently yields cleaner output after fewer review cycles, especially for creative or marketing content.
Of course, challenges exist:
- Prompt Engineering Skills: Effective use of LLMs demands new competencies around prompt construction, requiring upskilling and experimentation.
- Unpredictability: LLMs may “hallucinate” or introduce factual errors unless carefully guided and validated.
- Data Privacy: While post-editing typically occurs on internal content, prompt-based workflows may interact with cloud-hosted LLMs, raising privacy and compliance concerns.
Comparative Analysis: MTPE vs. Prompt-Editing
Dimension | Post-Editing (MTPE) | Prompt-Editing (LLM) |
---|---|---|
Customization | Limited; relies on static glossaries or retrained models | Highly customizable in real time through prompt updates |
Scalability | High for repetitive domains, less so for creative content | Equally scalable but excels with non-standard or creative content |
Linguist Role | Editor of machine text; reactive | Director of machine output; proactive prompt designer |
Error Propagation | Systematic errors are repeated unless manually fixed | Systematic errors can often be fully eliminated via prompt refinement |
Required Skills | Language editing, subject matter expertise | Prompt engineering, linguistic creativity, subject matter expertise |
Quality Consistency | Variable; depends on engine tuning and editor intervention | High with well-crafted prompts; requires LLM understanding |
As demonstrated, LLM-driven prompt-editing workflows not only rebalance the human-machine relationship but also empower translators to become active orchestrators of linguistic quality.
Implications for the Future of Localization
The rise of prompt-editing signifies more than simply a technological upgrade; it represents a shift in translational agency. Seasoned translators who once spent hours painstakingly correcting MT errors can now invest their efforts in designing effective prompts, guiding LLMs, and focusing on high-value activities like transcreation and cultural adaptation.
For localization project managers, the productivity gains and improved consistency offered by LLM workflows facilitate faster turnaround, better scalability, and the ability to respond to market needs with greater agility. However, it is essential to pair these gains with robust quality assurance practices and ongoing professional development to master the nuances of prompt engineering.
Crucially, as LLM technology matures, the role of human linguists will evolve rather than diminish. The future landscape favors hybrid approaches, where prompt-editing and post-editing coexist, tailored to distinct content types and project needs. Machine output must always be scrutinized for critical errors — but with intelligent prompt design, much of the low-value manual drift can be eliminated.
Conclusion: Adapting to the New Translation Workflow
The evolution from traditional post-editing to prompt-editing driven by LLMs marks a substantial leap in how the translation and localization industry operates. While post-editing still offers efficiency for formulaic and high-volume content, prompt-editing harnesses the contextual intelligence and flexibility of LLMs to raise the ceiling on translation quality and creativity.
For experienced translators and localization professionals, adapting to this new paradigm requires embracing continuous learning, acquiring prompt engineering skills, and adopting hybrid workflows that maximize both productivity and linguistic integrity. By leveraging LLMs as agile translation partners — rather than just as black-box engines — the industry stands at the precipice of a more collaborative, responsive, and high-value future.
Ultimately, as LLMs continue to reshape the translation process, those who master both post-editing and prompt-editing will unlock unprecedented levels of efficiency, quality, and client satisfaction, ensuring their continued relevance and leadership in the global language industry.
#post-editing #prompt-editing #machine translation #MTPE #LLM #large language models #translation workflow #localization #GPT-4 #Google PaLM #neural machine translation #translator productivity #translation quality #prompt engineering #language industry #AI translation #translation technology #hybrid translation #translation automation #linguist workflow #translation customization #translation consistency #quality assurance #translation innovation #future of localization #translation process #translation tr